Artificial intelligence is often presented as the key to success for many manufacturing companies. While the benefits are sometimes compelling, not all necessarily need them, many are not ready, and handing the reins to a robot raises concerns.
The confusion surrounding artificial intelligence (AI), Marc-André Bourque, CEO and founder of the firm Meliore, knows it well. This Laval firm specializing in artificial intelligence and quantitative analysis has assisted nearly half a thousand companies since 2010 and implemented projects with about a hundred of them.
“In general, people don’t know what AI is, what it can do, he notes. Is it important to integrate deep learning? Sometimes projects fail because there is not enough data, I tell them to have a programmer do it instead. »
That a dried cranberry and blueberry processor is a Quebec leader in Industry 4.0 makes people smile. But this is indeed the case of Fruit d’Or, a Plessisville company that took advantage of the fire at its factory in 2015 to focus on automation and become the “first processor of organic cranberries in the world”.
Armed with the data accumulated by its thousands of sensors and its automated processes, Fruit d’Or has embarked on an ambitious project: training an AI to manage all production.
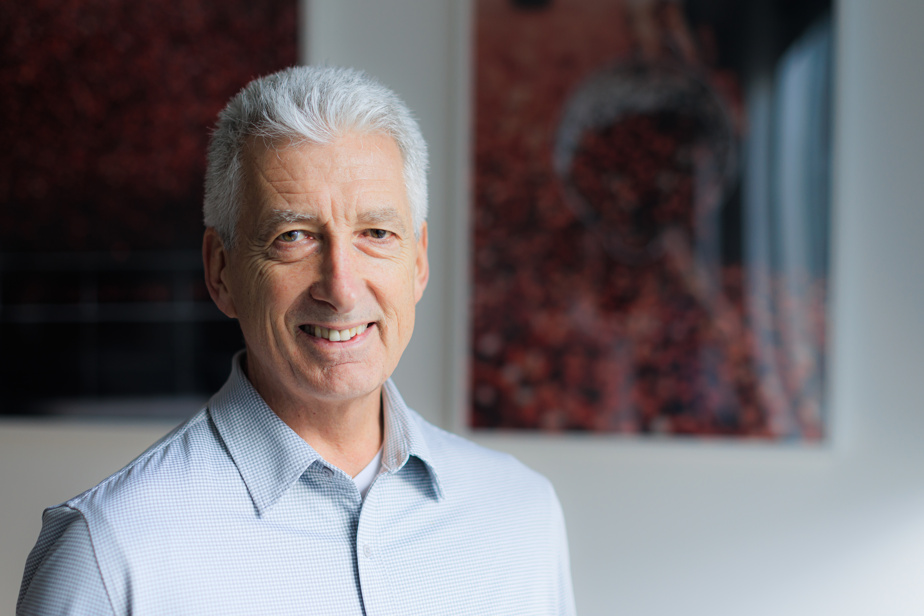
PHOTO EDOUARD PLANTE-FRÉCHETTE, LA PRESSE ARCHIVES
Martin Le Moine, President and co-founder of Fruit d’Or
The Press had enthusiastically reported on it in 2018. The project died about two years later, admits Martin Le Moine, president and co-founder of the company.
“We made the first 80% of progress which is the easiest, but the last 20% was going to cost us a lot of money. […] There have been many constraints. »
Turnkey solutions
It is not up to an SME like Fruit d’Or to invest millions to develop an AI solely for its needs, he believes.
“We’ve made progress, it has made us move forward, but I would expect a supplier of fruit dryers, which sells 20 a year, to do that. […] We have reached our fourth generation of optical sorter, the progress is incredible, but we are not the ones who are developing it. »
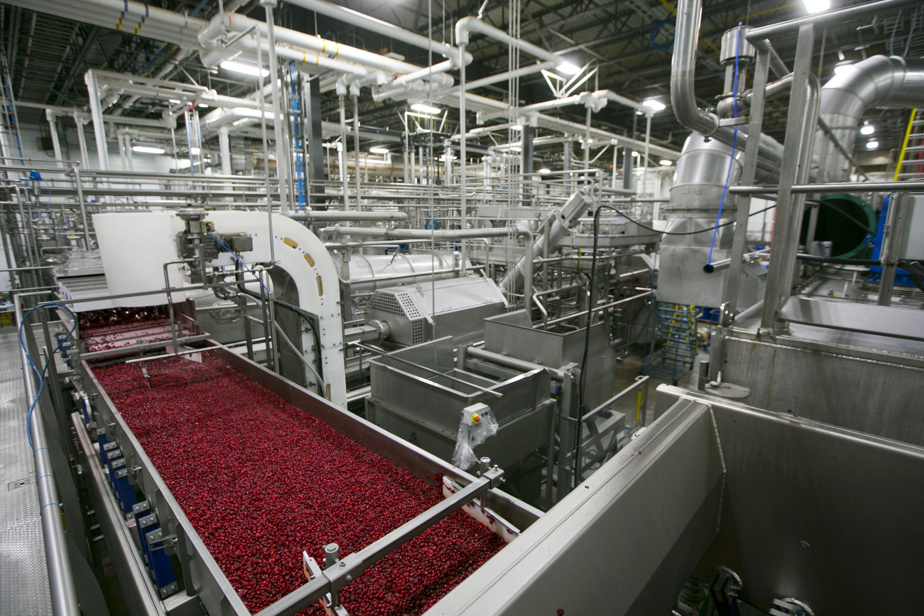
PHOTO FRANÇOIS ROY, LA PRESSE ARCHIVES
Fruit d’Or took advantage of the fire at its factory in 2015 to focus on automation and become the “first processor of organic cranberries in the world”.
Fruit d’Or uses advanced AI for its online marketing and advertising, he says, but these are still tools developed by giants like Facebook and YouTube.
In the manufacturing world, what I see is that these are often turnkey systems. Or we have machines that react. We are far from deep learning.
Martin Le Moine, President and co-founder of Fruit d’Or
The challenge for Quebec manufacturing companies is more down-to-earth, he argues. “The Quebec manufacturing sector, for the most part, is at the stage of automating, of collecting data. Before moving on to AI, we have a few years to go, we still have a lot of automation to do in Quebec. »
Quebec examples
Without aiming as high as Fruit d’Or, companies still manage to implement effective AI. The AI Activation program, created within the Mila Institute last June, has made it a specialty. For $5,000, for two to four months, we supported a dozen SMEs with experts who prepared a demonstration of the concept. “We have one foot in entrepreneurship and one in AI research, explains Michel Dubois, director of the Activation program. We ignite the spark, put a proof of concept in their hands to give them the desire to continue. »
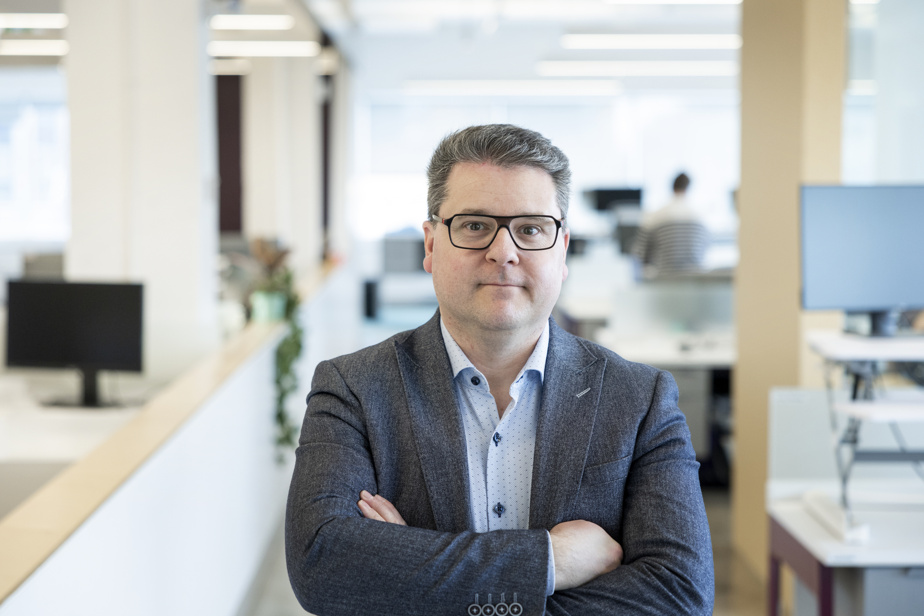
PHOTO MARCO CAMPANOZZI, THE PRESS
Michel Dubois, director of Mila’s AI Activation program
He gives four examples of such applications:
- Alloprof: A question-answer and text comprehension system.
- Dubois Agrinovation: Visual recognition makes it possible to find the spare part from a photo. “It used to take half an hour to search the catalog, now it takes a second. »
- CN: A tool summarizing the content of emails from customers and establishing statistics on the topics covered.
- GRICS: Analyzes for the company specializing in the development of software platforms in education to reduce school dropout by analyzing past interventions and their effect.
Mistrust
For Imran Ahmad, partner at Norton Rose and national head of technology law, it’s undeniable that business leaders are wary of AI. “There is a certain hesitation, people are afraid of changing everything overnight. The solution is to gradually implement certain less critical functions. “In a manufacturing environment, there is an element of risk as soon as something unexpected happens. We must have enough data to make solid proofs of concept. »
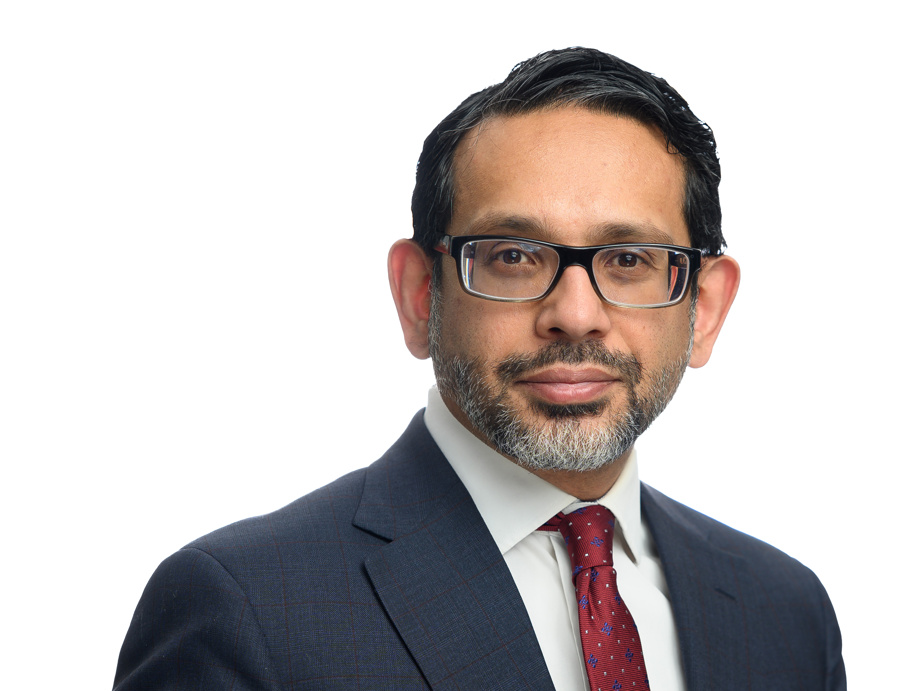
PHOTO [email protected], PROVIDED BY NORTON ROSE
Imran Ahmad, Partner at Norton Rose and National Head of Technology Law
As an interesting prospect, he believes that AI can give smaller companies the opportunity to compete with larger ones. “It levels out, we don’t have to hire as many people or build major infrastructure. Incorporating AI into small businesses can happen very quickly. »
One of the most profitable and least risky areas of AI implementation concerns equipment maintenance planning, notes Françoys Labonté, CEO of the Montreal Computer Research Center (CRIM). “The performance of the AI is equivalent or better, and we don’t have to worry about it screwing up production. He too favors a gradual approach with most SMEs. “We will test classical approaches, data science, good old linear regressions, and then we can have a machine learning project. It’s not perfect, but it’s iterative. »
Deep learning isn’t always necessary, he reminds us. “There is a cost. Training a system for two weeks, GPU over GPU, to improve a system by 0.5%, is it worth it? »
Three tips for implementing AI
1. Ethics
The use of public online tools, for example for translation or storage, must also be done with caution if confidential documents pass through them. Ethics in AI also means ensuring that the system does not have biases or can be used for malicious purposes. “I have already done projects that could be qualified as unethical, for example to quantify the work of a human on an assembly line, says Marc-André Bourque, from Meliore. It was working, it was cool, but I stopped it. »
2. Data
The key to deep learning is having enough good quality, standardized data to train the AI on. If the company has not accumulated them itself, it is sometimes risky to use external data sets. “We will train a system whose source we do not control, which will have to be supplemented, sometimes corrected or enriched with synthetic data”, explains Michel Dubois, director of the Activation program.
3. Iterations
Rather than aiming for large-scale implementation from the outset, it is better to select certain activities. “You have to try as much as possible to do short iterations and impact analyses, to evaluate the advantage of a good answer and the disadvantages of a bad one,” explains Mr. Dubois. It’s a bit like a new employee: we help him, we watch him a little, we give him less critical tasks at the beginning. »
Learn more
-
- 15%
- Percentage of Quebec companies that have integrated AI into one of their activities
survey published in May 2022 by the Order of Certified Human Resources Advisors (CRHA)